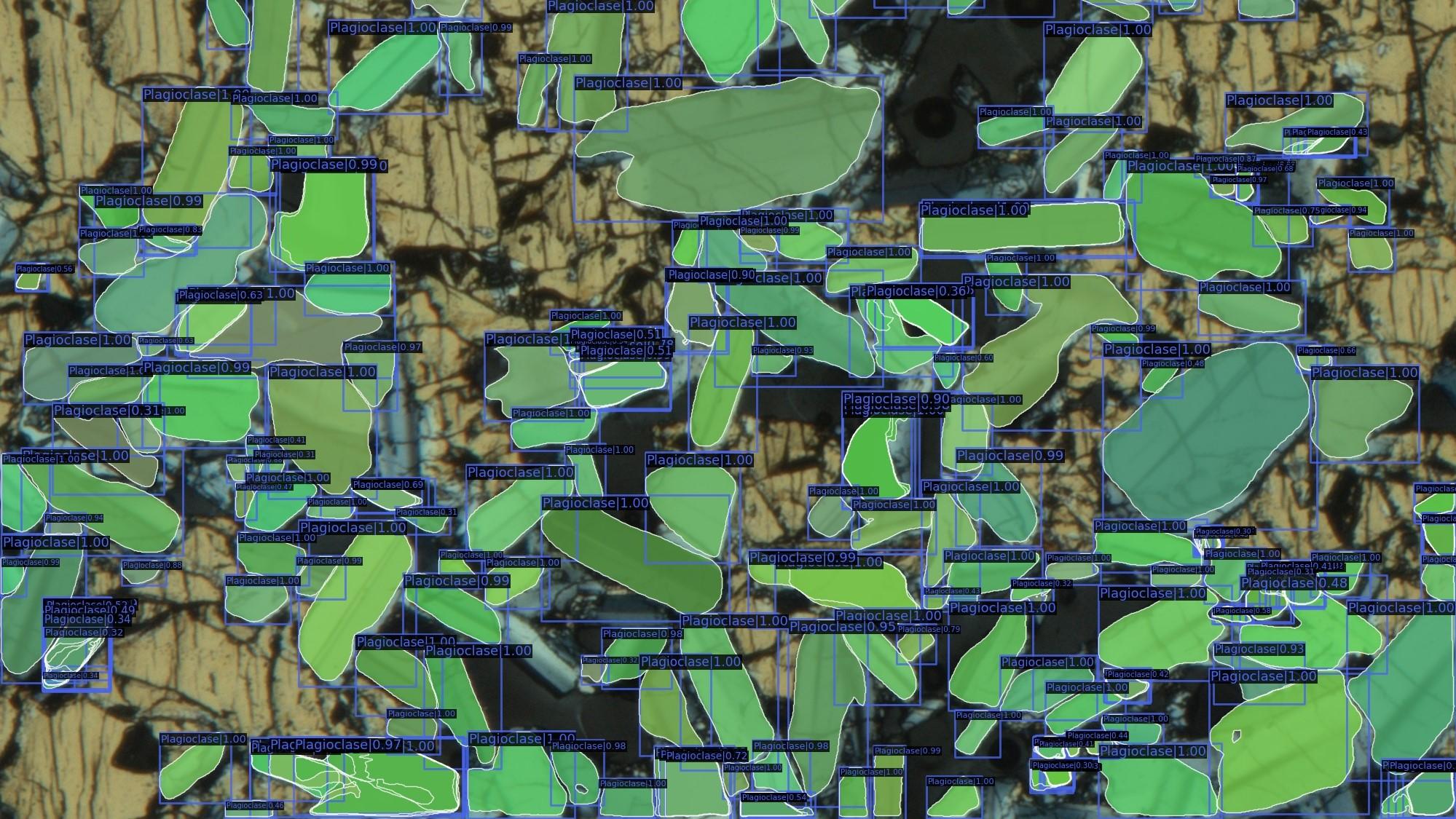
Submitted by Dr C.M. Martin-Jones on Tue, 25/04/2023 - 09:11
Plagioclase feldspars are one of the most common and abundant mineral groups in Earth's crust — and that means they are also one of the most frequently studied minerals. Clues locked away in these crystals can provide information on a range of broad geological questions from, for instance, the processes that triggered a volcanic eruption or the route that magma took to the surface.
Traditionally, petrologists have extracted clues from plagioclase feldspars by measuring crystal shapes and sizes under the microscope. It’s a tried and tested method, but one that has proved fairly labour-intensive because it involves manually tracing out crystals from a microscope image. That could, however, be set to change, because researchers from Cambridge Earth Sciences have now developed a deep learning tool that automates measurements of plagioclase feldspars.
PhD student Norbert Toth developed the method which reduces data collection time by roughly 90% whilst maintaining the accuracy of traditional methods. “My aim is to attempt to significantly speed up methods of data collection or phase identification in thin sections,” said Toth, who will be presenting the research at EGU23. He adds, “I hope this tool will be of use to people across the department, who are doing a whole range of fantastic research.”
Toth trained commercially successful deep learning models to find crystals and trace their outline in microscope images. Plagioclase feldspars are distinctive to the human eye — the zebra-striped twinning making them easily recognisable. But, for those who have previously attempted to train machine learning algorithms, this twinning can complicate straight forward automation. Previous approaches often failed to tell the crystals that are touching apart from each other.
By overcoming this issue, the new method can give the exact pixel-to-pixel location and size and aspect ratio measurements. Toth has tested the approach on 27 samples from Iceland, segmenting over 38,000 crystals in less than two days of computing time. “The results we get are essentially just what people would get from manually tracing crystals, but, thankfully, much faster and without any manual work,” said Toth.
Toth, N., & Maclennan, J. (2023). A Deep Learning Enabled Approach for Igneous Textural Timescales (No. EGU23-9289). Copernicus Meetings.